Lasso Regression and Its Use in Data Science
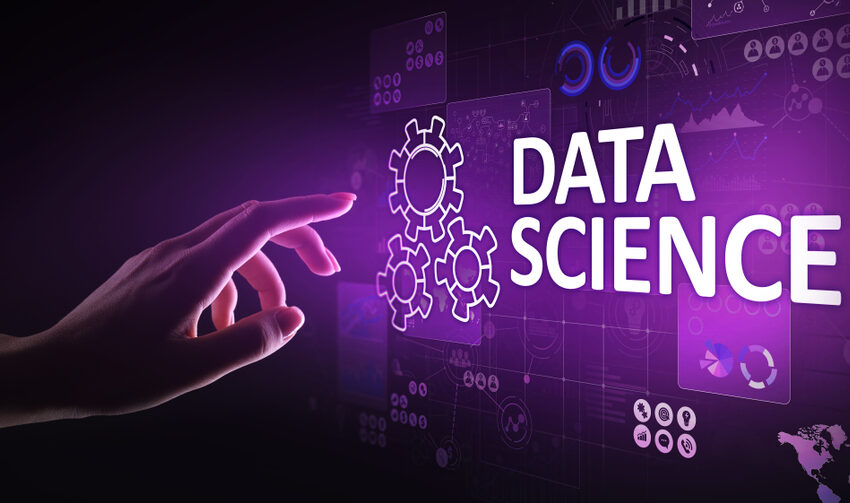
In the ever-evolving field of data science, selecting the right tools and techniques is crucial for building robust predictive models. One such powerful tool is Lasso Regression, which can enhance model performance and interpretability by addressing overfitting and feature selection challenges. Understanding Lasso Regression and its applications can significantly benefit anyone looking to excel in data science. Enrolling in a data science course in Mumbai can provide the necessary expertise and practical experience for those eager to dive deeper into this topic.
What is Lasso Regression?
Lasso Regression, abbreviation for Least Absolute Shrinkage and Selection Operator, is a specific type of linear regression that incorporates regularization to prevent overfitting and enhance model simplicity. Unlike traditional linear regression, which minimizes the sum of squared residuals, Lasso Regression adds a penalty which is equivalent to the absolute value of the coefficients’ magnitude. This penalty term encourages the model to shrink less important feature coefficients to zero, effectively performing feature selection.
A data science course covers the theoretical foundation of Lasso Regression, explaining how adding the penalty term helps mitigate overfitting by simplifying the model. This ensures that the model generalizes unseen data better.
The Role of Regularization
Regularization is a methodology that penalizes the complexity of the model to prevent overfitting. In Lasso Regression, the regularization parameter (lambda) controls the strength of the penalty. More coefficients are shrunk to zero in a simpler, less feature-rich model when the lambda value is larger. Conversely, a smaller lambda allows more features to remain in the model, potentially capturing more complex relationships but increasing the risk of overfitting.
By taking a data science course in Mumbai, students can gain hands-on experience with regularization techniques. Mumbai’s vibrant tech ecosystem offers numerous opportunities to work on real-world datasets, applying Lasso Regression to balance model complexity and performance.
Feature Selection with Lasso Regression
One of the key advantages of Lasso Regression is its ability to carry out automatic feature selection. By shrinking some coefficients to zero, Lasso Regression effectively removes irrelevant or redundant features from the model. This improves model interpretability, reduces the risk of overfitting, and enhances computational efficiency.
A data science course provides practical training on implementing Lasso Regression for feature selection. The course includes coding exercises and projects demonstrating how Lasso Regression can identify the most important predictors in a dataset, making it easier to build parsimonious and interpretable models.
Use Cases of Lasso Regression
Lasso Regression is widely used across various industries and applications due to its versatility and effectiveness. Some common use cases include:
- Predictive Modeling in Finance: Lasso Regression helps in selecting the most relevant financial indicators for predicting stock prices or credit risk. Analysts can build more accurate and interpretable models by focusing on the most important features.
- Healthcare Analytics: In healthcare, Lasso Regression is used to identify key predictors of patient outcomes from many potential risk factors. This aids in developing targeted treatment plans and improving patient care.
- Marketing and Customer Insights: Marketers use Lasso Regression to determine which customer attributes are most predictive of purchasing behavior. This information can guide targeted marketing campaigns and optimize resource allocation.
- Environmental Science: Lasso Regression helps in modeling complex relationships between environmental variables and outcomes, such as predicting air quality based on meteorological data.
Students can explore these use cases through hands-on projects and case studies by enrolling in a data science course in Mumbai. The course curriculum is designed to provide practical insights into how Lasso Regression can be applied to solve real-world problems.
Conclusion
Lasso Regression is a powerful tool in the data scientist’s toolkit, offering the dual benefits of regularization and feature selection. Its ability to prevent overfitting and simplify models makes it particularly valuable in the era of big data, where datasets often contain numerous features with varying degrees of relevance. For those looking to master Lasso Regression and other advanced techniques, enrolling in a data science course is a great step. These courses provide comprehensive training on the theoretical and practical aspects of Lasso Regression, equipping students with the skills needed to excel in the dynamic field of data science.
Business Name: ExcelR- Data Science, Data Analytics, Business Analyst Course Training Mumbai
Address: Unit no. 302, 03rd Floor, Ashok Premises, Old Nagardas Rd, Nicolas Wadi Rd, Mogra Village, Gundavali Gaothan, Andheri E, Mumbai, Maharashtra 400069, Phone: 09108238354, Email: enquiry@excelr.com.